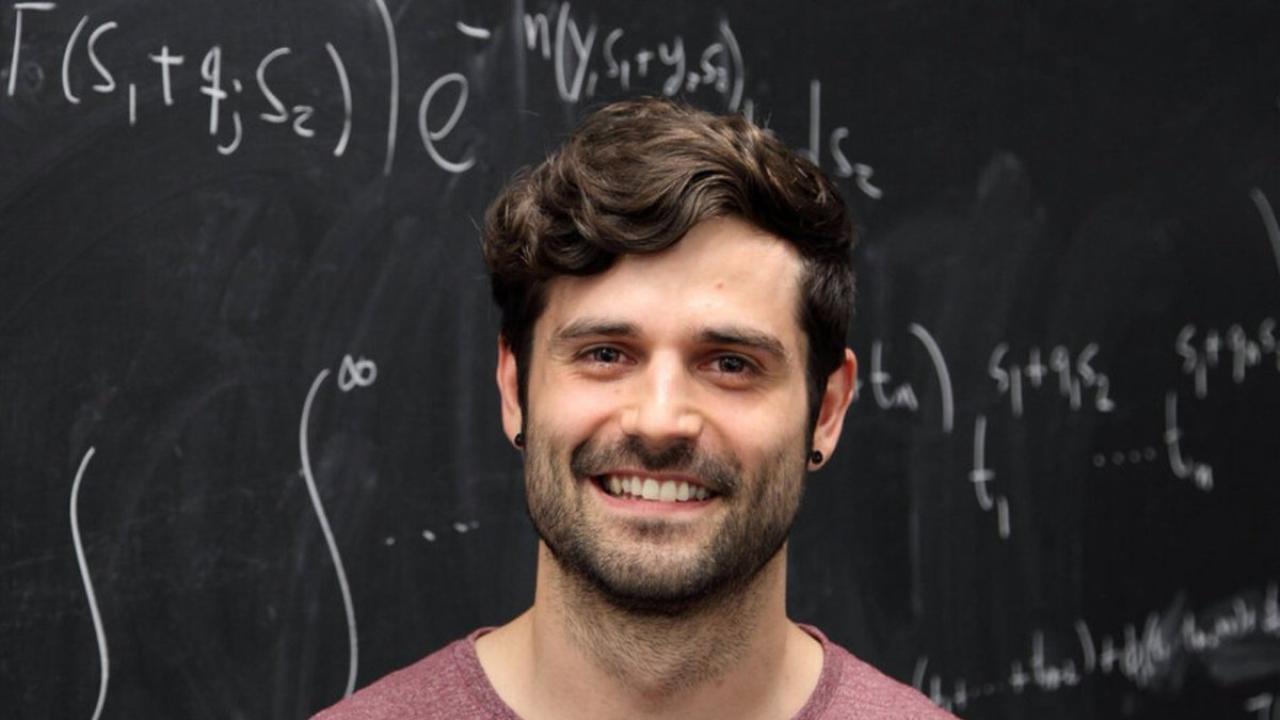
ICTP researcher Jean Barbier has been awarded a prestigious European Research Council Starting Grant to support his research in statistics for machine learning. A statistical physicist with a strong interest in mathematics and data science, Barbier works in ICTP's Quantitative Life Sciences section.
The ERC grant will fund a five-year multidisciplinary project that will tackle questions concerning machine learning and neural networks using high-dimensional statistics, that is, the statistics needed to understand modern information processing and inference systems, and information theory.
"Machine learning and neural networks are at the root of many technological applications, such as self-driving cars, natural language processing, and image recognition," says Barbier. "These are versatile and powerful algorithms, but they are extremely complex. The main problem in this field nowadays is that we miss a strong theoretical explanation of why they work so well. The type of neural networks that scientists are able to mathematically analyse are somehow oversimplified with respect to the realistic ones that are used in practice by engineers in a multitude of technological applications. There is a huge gap between the two."
The goal of the project is to provide better statistical tools in order to model more "realistic" neural networks that are used on a daily basis in modern technologies, and to precisely quantify the performance of such algorithms.
The title of the project, CHORAL, comes from "Computational Hardness Of RepresentAtion Learning". Representation learning refers generically to the set of actions and techniques of parameters tuning, performed by virtual machines, that allows a system to automatically classify raw data. "In a machine learning process, what we want is that the machine creates an internal representation of some complex input data," says Barbier. "Once the machine has been trained and has learned from data, it is then able to generalize, to process new data independently and efficiently using its own representation of it."
Studying the computational hardness of such systems' learning then means quantifying and comparing two kinds of costs. The first is the so-called "information theoretic cost" of learning the neural network, or "statistical cost".
"Given a mathematical model of a neural network, and a certain model for the data that you use to train it, we want to quantify how much data you need for the neural network to produce correct predictions once it has been trained, regardless of any computational limitation," says Barbier. "What is quite surprising is that there is a fundamental threshold below which it is impossible for the neural network to correctly predict. There is simply not enough data, not enough information for it to generalize on new data." This threshold is not a technological barrier, but rather a fundamental one. It is physically of the same nature of thresholds that separate different states of matter, i.e. phase transitions. In this context, the perspective of physics, and in particular of statistical physics, really gives a valuable contribution to the field.
The second cost, that needs to be compared to the statistical cost, is the so-called “cost of efficiency”. This is the amount of input data necessary for a virtual machine to correctly process new data when trained within a reasonable time frame. "Adding the requirement that the learning must be rather fast creates a second threshold: this actually means that usually more data are needed compared to what is required from the statistical viewpoint," says Barbier. "What I really want to achieve with this research is setting these boundaries, these so-called 'statistical-to-computational gaps' appearing when there is a discrepancy between these two costs. From those, it is then possible to derive benchmarks, and eventually to describe the phenomenology in the representation learning of neural networks that are as close as possible to those used nowadays in realistic machines."
Barbier is one of the 397 early-career researchers who won European Research Council (ERC) Starting Grants this year. Starting Grants are worth on average €1.5 million and will support talented younger researchers in launching their own projects, form their teams and pursue their best ideas. Barbier is looking forward to taking advantage of this opportunity and is hoping that some of this money will fund at least four post-doctoral fellows to join him on this exciting project. "I am also hoping that this project will trigger some activity and attract a spotlight on the recently established Data Science and Artificial Intelligence Institute in Trieste," says Barbier.
His project will likely have a direct practical impact in the fast-growing field of machine learning. "By understanding more realistic models of neural networks and their fundamental limits, researchers would then be able to test practical algorithms against these limits to see if they are good ones or not," says Barbier. "In this way engineers won't waste their time on trying to improve a certain algorithm if they know that it is already optimal."
On the other hand, this research is also promising on a more fundamental level. "An analogy that I like is that between what I am doing and the birth of information theory thanks to the work of Claude Shannon," says Barbier. "Information theory stemmed from engineering questions, such as how to communicate data efficiently at high rates, and Shannon gave us the tools to understand that there is a fundamental limit to communication and to data compression. I am really using the same kind of approach when analysing learning: I want to understand the fundamental limits in terms of data needed to learn, and to do it efficiently."
--- Marina Menga