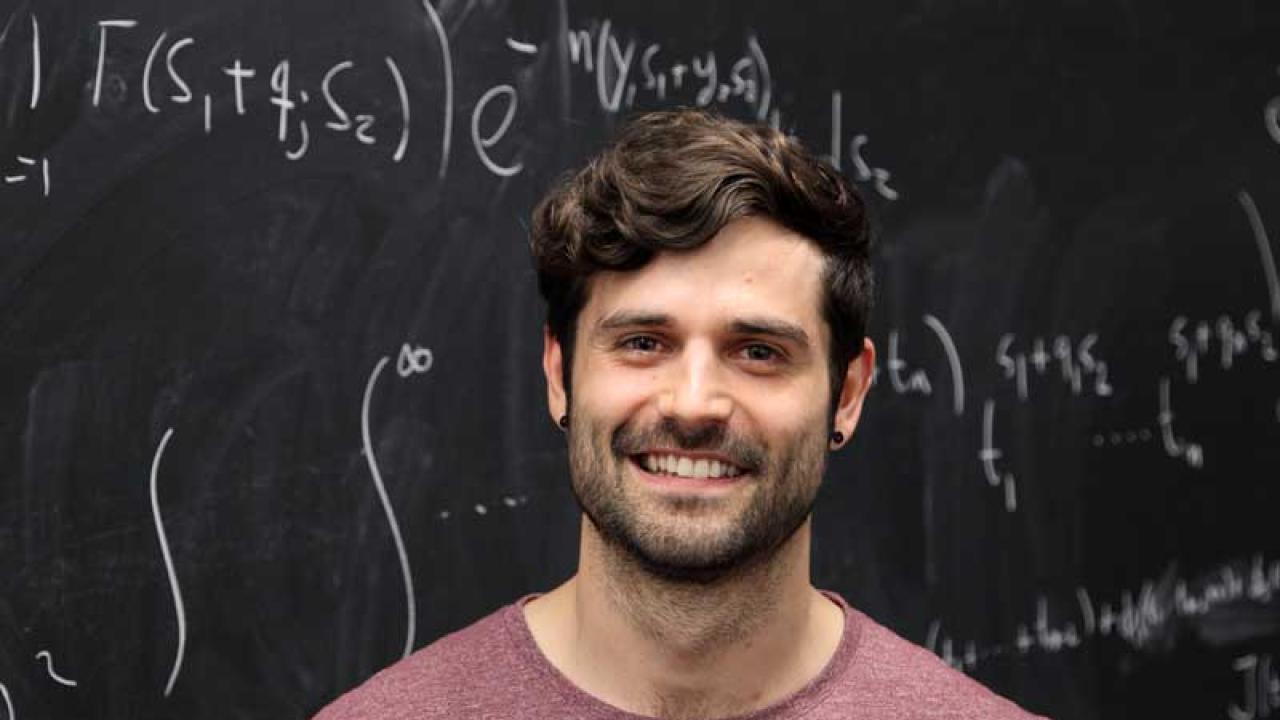
What do you do when you have a lot of data points, and you’re trying to make sense of what they tell you? Which data points are noise, meaningless information and fluctuations, and which represent the phenomenon you’re interested in knowing more about? A model is a story built of mathematics, a proposed explanation of patterns in the data and what is making them, and models are built into all sorts of technology and processing. The tricky step is to figure out what is noise and what is data, inferring backwards when you know something about the model that helped generate the data.
The generalized linear model is a very common way of generating data, a flexible model that is used in signal detection and processing in many fields, including digital communications, developing medical imaging techniques, or studying machine learning. Separating noise from signal produced through a generalized linear model (GLM) is a common challenge, and one of ICTP’s recently hired researchers, Jean Barbier, has analyzed the fundamental limits of reconstructing data from the GLM. His work clarifies the overarching characteristics and power of algorithms used for data reconstruction. The results were recently published in the journal Proceedings of the National Academy of Sciences.
“The most interesting part of this result, to me, is the generality of it, how we’ve outlined a single framework that can be applied to lots of fields,” says Barbier. “The GLM is now used by the machine learning, signal processing, and statistical physics communities among others, it’s commonly used. But a lot of the approaches to data reconstruction are not mathematically rigorous, which does not mean not exact.” Barbier and his collaborators managed to establish the limits of reconstructing data from the GLM in a mathematically rigorous way. Coming from a physics background, the team used physics methods and mathematical proofs to establish precisely when it’s possible to use an algorithm to sort signal from noise and when it is not.
The new limits help provide a rigorous way of assessing specific algorithms’ success, but go beyond the binary of whether a specific algorithm works or doesn’t work. The paper identifies several phases or levels of functionality and success. “It’s actually very similar to the phases of matter in physics,” says Barbier. “It’s a bridge between the physics of data and the physics of matter.”
The different phases of algorithmic behavior include the ‘impossible phase’ where there is no way for any algorithm, efficient or not, to reconstruct the signal and extract the desired information. There is just too much noise obscuring the data. The ‘hard phase’ is where an algorithm for finding the signal exists, but no efficient algorithm can- a huge amount of computing power would be required. The ‘easy phase’ is where a well known efficient algorithm can reconstruct the signal, without a big expense of computational hours. As with phases of matter, there could also be other phases of ‘algorithmic phases’ beyond these three, Barbier says.
As a new member of the Quantitative Life Sciences section at ICTP hailing from France, Barbier’s research interests provide more examples of interdisciplinary applications of ideas from physics. “I would say I’m a physicist who works in information theory and statistical learning,” Barbier says, “I’m a physicist in how I conceive toy models, not being stopped by mathematical details when I approach problems, but I’m interested in applied machine and statistical learning questions.” Barbier is also interested in mathematical proofs as a tool for research. “When you prove theorems, you understand the physics much better.”
Barbier is pleased with his new ICTP affiliation. “Here you can do top class science and can also have an impact away from the research, supporting and connecting with scientists who have fewer opportunities and are just as bright and motivated as any scientist in richer countries.” Barbier is currently working with new colleagues in Trieste to organize a workshop aimed at gathering young mathematicians, physicists, and information theorists, to build the international community of scientists working at these junctures.
The new paper is a great way to mark the beginning of Barbier’s time at ICTP. “We take a very general and hard-to-understand model and establish some fundamental limits on whether the signal can be reconstructed from the data,” says Barbier. “We’re hoping this stands as a reference paper, a benchmark guide to using this tool.”
---- Kelsey Calhoun