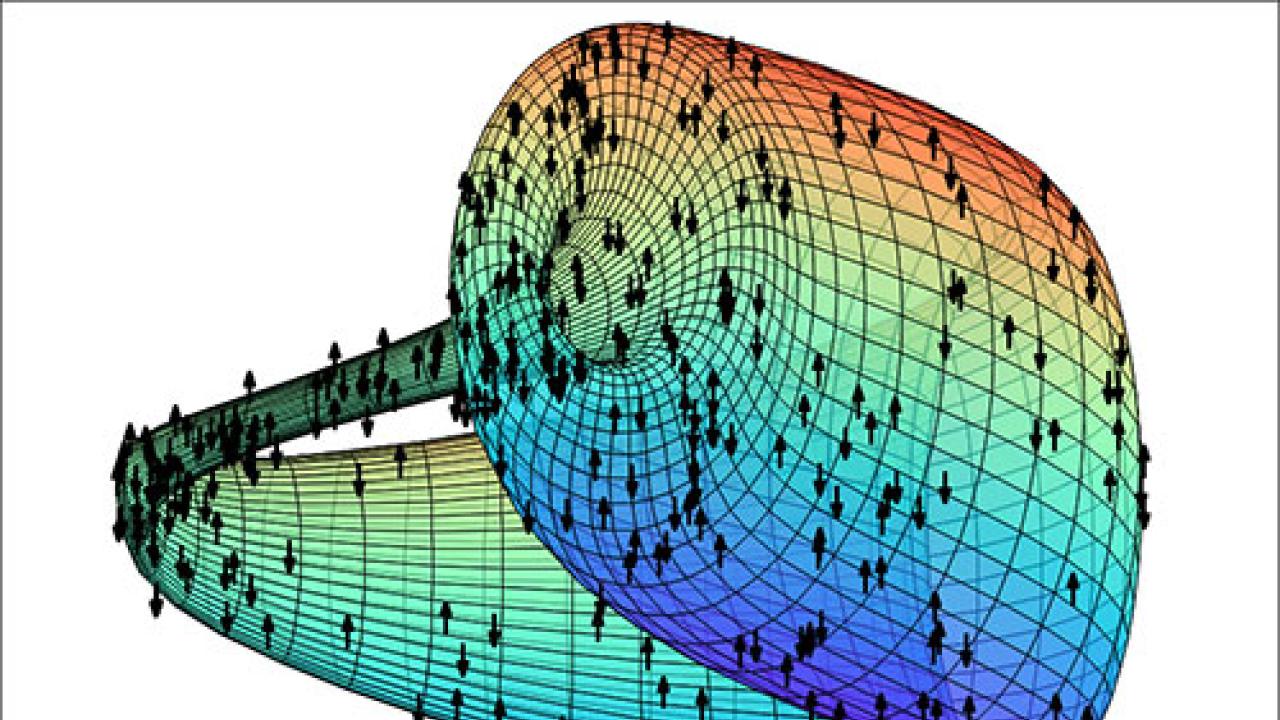
Information is encoded in data. This is true for most aspects of modern everyday life, but it is also true in most branches of contemporary physics, and extracting useful and meaningful information from very large data sets is a key mission for many physicists.
In statistical mechanics, large data sets are daily business. A classic example is the partition function, a complex mathematical object that describes physical systems at equilibrium. This mathematical object can be seen as made up by many points, each describing a degree of freedom of a physical system, that is, the minimum number of data that can describe all of its properties.
An interdisciplinary team of scientists from ICTP and the International School for Advanced Studies (SISSA) showed that such a massive collection of data can be combed through, bringing out fundamental physical properties of an unknown system.
These results were highlighted in a paper just published in Physical Review X, introducing a new data-based viewpoint on phase transitions. The team showed that a generic statistical property of large data sets that describe a broad range of physical systems at equilibrium can in fact reveal the occurrence of a phase transition.
The authors of the paper, coordinated by Marcello Dalmonte, a researcher in ICTP’s Condensed Matter and Statistical Physics Section and SISSA collaborator, come from different backgrounds. Tiago Mendes, a former postdoctoral fellow at ICTP and now at the Max Planck Institute for the Physics of Complex Systems, in Dresden, Germany, works mainly in numerical methods applied to statistical mechanics. Alex Rodriguez is a chemist, previously working at SISSA and now at ICTP, who works in the implementation of complex system algorithms and the development of machine learning methods. Xhek Turkeshi, a PhD student at SISSA, works mostly in statistical physics.
The collaboration started when Rodriguez arrived at ICTP’s Condensed Matter section two years ago as a Boltzmann senior fellow and was interested in knowing what his colleagues were working on. “It all started as a kind of informal chatting with all the people in the section,” says Rodriguez. “I was an independent researcher, I didn't have a group, so I wanted to collaborate with the other researchers in the field.” Before the outbreak of the Covid-19 pandemic, informal chats and random encounters at ICTP’s bar, terrace and corridors were often the perfect start of new projects and new research. “We realized that people working in statistical mechanics and many body physics are now looking more frequently to these kinds of problems,” adds Mendes. “They are looking for ways to apply machine learning methods to statistical mechanics problems, so we thought we would try and follow this common interest in the field.”
At the beginning, however, things were not so straightforward in terms of team-work, as coming from different backgrounds affected the capacity of effective communication between them. “I remember that, at the beginning, we didn't even have a clear idea of what to expect from the data we were generating, so it was really exploratory research at first,” says Turkeshi. “What really helped us in the end was to have a timescale over which to close the gap between the work done by Alex with data science methods and our perspective which was at first totally different.”
Then the pandemic started, adding to these difficulties the disadvantages of working from home, as work had to move online, and science discussions started to happen on Zoom. “The paper was written during the lockdown and it was much more difficult when you could not meet anymore at ICTP and everybody was at home,” says Mendes. “There was a very difficult topic to address at a certain point and we decided to meet at a bar to discuss in person. I think that by meeting online you cannot really find these kinds of creative ideas, it's really difficult. You need personal interaction to generate something new.”
The researchers focussed on a generic statistical property of the data sets, called the intrinsic dimension. The simplest way to describe this property is as the minimum number of variables needed to represent a given data set, without any loss of information. “Take, for example, all the people around the world,” explains Rodriguez. “That is a data set by itself. Now, if you want to specify the position of the people around the world, in theory, you would need the coordinates of all their positions in space, that is, three data for each person. But since we can approximate the Earth as a bidimensional surface, we will only need two parameters, that is, the latitude and the longitude. This is what intrinsic dimension is: if the data set was humanity then the intrinsic dimension would be 2, not 3.”
In the more theoretical context of statistical systems, the paper shows that this property of intrinsic dimension can reveal collective properties of partition functions at thermal phase transitions. This means that, regardless of what system is under consideration, the data can show if and when that system is undergoing a phase transition. The team has developed a theoretical framework to explain why generic data exhibit such a “universal” behaviour, common to a broad range of different phase transitions, from melting ice to ferromagnets.
“The work introduces a new viewpoint on phase transitions by showing how the intrinsic dimension reveals correspondent structural transitions in data space,” say the scientists, “when ice melts, its data structure does as well.”
“What is really new in this work is that raw data mirror the physical behaviour of the systems we consider,” says Rodriguez. “And that is important because it allows us to analyse a system without knowing the physics underlying it. Just having the data can tell us if there is a transition happening in the system or not, without even knowing what kind of transition it is.” “We could say that this method is completely agnostic,” adds Mendes. “You don't need to know a priori all the parameters of the system; you just work with raw data and see what comes out of them.”
After the interesting results obtained in this research, the team plans to continue working together in the same direction, broadening their field of analysis. They are already working on a second paper, focussing on the so-called “quantum phase transitions”, that is, quantum systems where phase transitions happen at a temperature equal to zero and are induced by external parameters, like the magnetic field.
In terms of applications of these findings, the possibilities are many – from experiments with computer simulations of quantum systems to more fundamental branches of physics, such as quantum chromodynamics, that could also have an impact on nuclear physics. “An interesting possibility of application is in the use of statistical physics techniques to understand machine learning,” says Rodriguez. “In this kind of research, that goes from quantum computing to the study of neural networks for example, phase transitions are very often involved and we could try to use our method to tackle all these kinds of different problems.”
The article, titled "Unsupervised Learning Universal Critical Behavior via the Intrinsic Dimension", is open access and is available at https://doi.org/10.1103/PhysRevX.11.011040.
---- Marina Menga