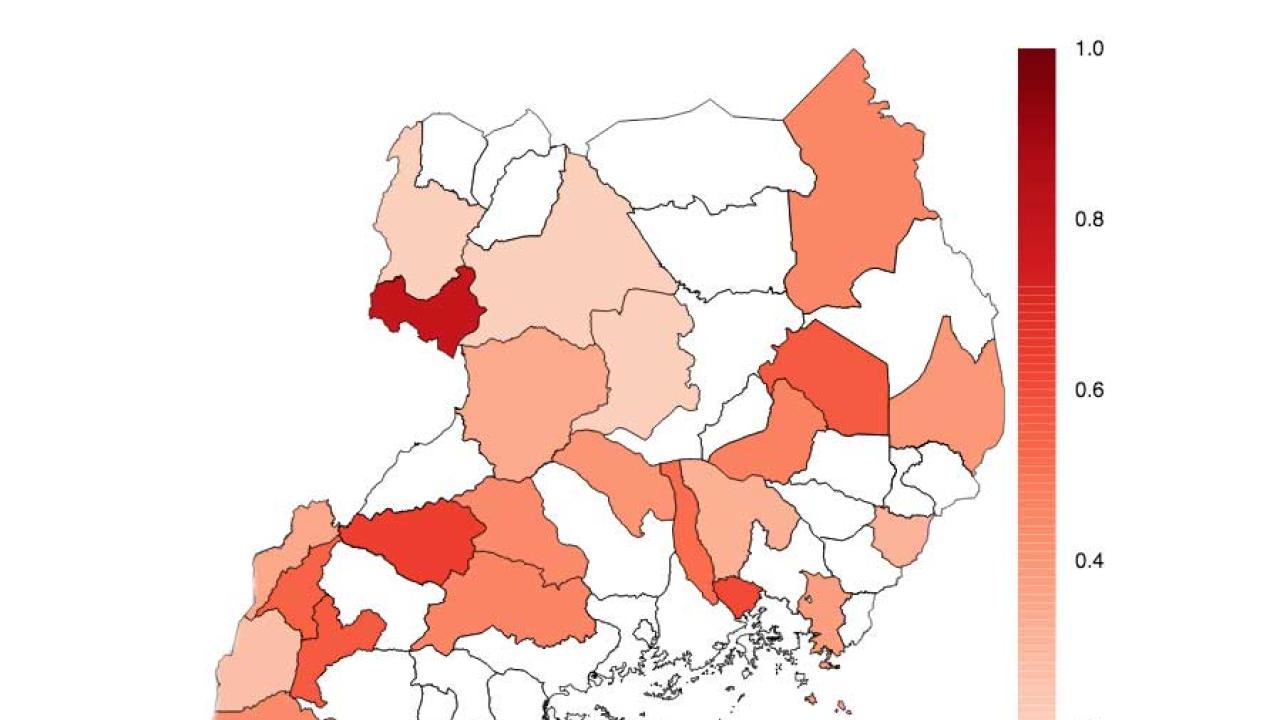
Weather and climate are underpinned by complicated chaos, making long term predictions very difficult. Adding another layer of complexity and chaos in the form of the life cycle of mosquitos makes forecasting even harder, but that is what ICTP scientist Adrian Tompkins and his collaborators did in a pilot system of predicting malaria outbreaks across Uganda.
Results of the pilot study have been published in the 8 January issue of GeoHealth.
"The idea of the research was to attempt to predict anomalies in malaria transmission at the district or local scale in Uganda," explains Tompkins. "We used weekly and seasonal predictions of temperature and rainfall from the European Centre for Medium Range Weather Prediction (ECMWF) to drive a mathematical model of the disease transmission developed at ICTP. It is the first time that such an attempt has been carried out at the subnational scale."
Computer models of climate systems are starting to be put to work in the public health sector, and this system is a prime example. If health departments and hospitals knew when and if a malaria outbreak was due to hit far enough in advance, preparations could be more targeted, cost-effective, multi-pronged, and overall more successful at taking care of patients and communities.
The number of malaria cases in humans depends on mosquitos, which carry malaria and infect those they bite. The population of mosquitos, in turn, depends on a large number of climate-related factors, as they need a certain temperature range and standing water to lay their eggs in. How land is used, how human populations move, altitude, recent storms, and many other factors can also affect mosquito populations and their spread of malaria.
The new system applies a customized model to the problem of malaria prediction, combining existing models for monthly and seasonal forecasts with a model of mosquito population cycles to predict infection rates. This malaria early warning system is one shown to be useful at predicting at a regional scale, not just a national scale. Data from three sites in Uganda above 1100 meters were used to test the effectiveness of the system; at that altitude, climate variability is a stronger driver of malaria transmission. The success of the system was measured as the predictions of transmission compared to measured transmission four months later.
"Forecasting malaria anomalies accurately is notoriously difficult," says Tompkins. "Climate variability is only one of many factors that determine variations in malaria rates, and moreover, health data records are often relatively short and can suffer from data uncertainties. One of the things that surprised us in this study is that using high quality data from sentinel health clinics, the system was able to predict differences in malaria between some regions up to three or four months ahead."
Tompkins continues: "The paper uses a simple economic analysis to try to guide decision makers as to when it would be beneficial to implement interventions. We feel such an analysis is a crucial first step to help integrate climate information into the health planning procedures."
Tompkins cautions that the system is still far from perfect: at the district level, the forecasts were only effective in half of the regions in Uganda, and further research is required to better understand data uncertainties and improve the forecasting system. He says that a proposal is currently underway to the European Union to address these concerns and look at how best to "operationalize" the pilot system.