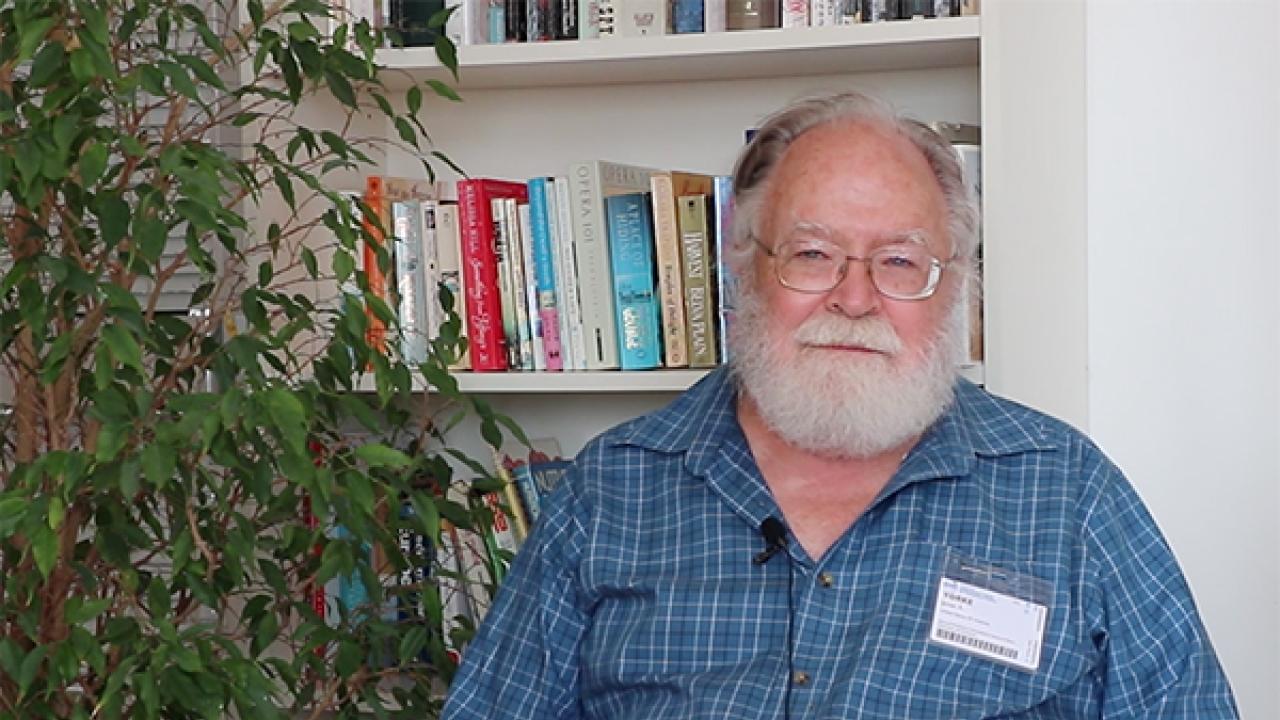
Mathematician and physicist James Yorke, known as the "father of chaos", was recently at ICTP to participate in the Advanced Workshop on Nonequilibrium Systems in Physics, Geosciences, and Life Sciences.
Yorke is a Distinguished University Research Professor of Mathematics and Physics and former chair of the Mathematics Department at the University of Maryland, College Park. He and a co-author are credited with introducing the mathematical term "chaos" in a 1975 paper. ICTP asked Yorke about the meaning of chaos and its applications; the interview is below.
Professor Yorke, while you are here in Trieste for the Advanced Workshop, you gave a talk titled “Low dimensional examples of Multi-Chaos: non-homogeneous high -dimensional chaos”. What is chaos and multi-chaos?
Chaos is a term we use to introduce scientists to ideas that everybody knows. It’s a simple and intriguing idea that can apply to everyone and give insights into very complicated situations. Everybody knows that little changes in your life can make huge impacts (your car gets a flat tire and you run late, you get fired and you become poor). Chaos is about situations that are predictable in the short run but unpredictable in the long run because little changes have big outcomes. Multi-chaos, I like to put this way: chaos is when you play billiards on a table. The billiard balls bounce around, hit each other and can diverge in different directions. That’s chaos. But if the balls bounce off the table, that becomes chaotic in more directions: multi-chaos.
Before 1975, people observed these phenomena but didn’t have the terminology to describe them. There is no mention of chaos in the engineering or mechanics books of the 1970s. But after you tell physicists and mathematicians and engineers what they should be looking for, now everybody knows about chaos in their own systems.
Maxwell gave an interesting example to describe chaos: if you have a leopard and you know where every atom in its body is and the way it is moving, within a tiny fraction of a second they will all bounce against each other and you won’t know anymore. But the leopard will not change its spots: so, after all, the chaos is within certain bounds. The chaos is bound, that’s the standard meaning and that’s the way it is in science too.
Talking about chaos, you often come across this idea of the “butterfly effect”. What is it and why has it become so popular?
A butterfly flapping its wings causes some little change and that tiny change can imply bigger changes, maybe not all the time, maybe rarely, but it can even form a tornado somewhere on earth. But let me tell you something on behalf of the butterfly that nobody really mentions: a butterfly flapping its wings can also prevent a tornado to form, or it might shift a tornado from Texas to Florida.
But I don’t think Edward Lorenz was the first one to come up with this idea, because I can go back to Benjamin Franklin, who became rich publishing his almanac, which was filled up with sayings, including “For want of a nail the shoe was lost": For want of a shoe the horse was lost. For want of a horse the rider was lost. For want of a rider the message was lost. For want of a message the battle was lost. For want of a battle the kingdom was lost. And all for the want of a horseshoe nail.
Here you see not only that a tiny effect has big effects, but it also shows how this can grow. Maybe each step is unlikely, but with enough nails and enough shoes these things can happen. It’s all about little changes growing and growing in size.
In different fields (meteorology, climate, brain functions, economy), many phenomena are still unpredictable; what is the problem?
Let’s think about the billiard. If you know the starting condition of a ball hit on a billiard table, you can predict where it will go. If you add many more balls, the problem becomes soon much more complicated. That’s the basic phenomenon. If you have a certain uncertainty in the state of the weather worldwide, you don’t really know the temperature in every point for example, this goes into your prediction: depending on your model, how accurate and detailed it is, in two days your uncertainty would have been doubled and so on. You can’t measure the temperature in the Trieste area with infinite accuracy. You have a limited accuracy and limited knowledge (and too complicated systems).
Research published recently in Physical Review Letters has demonstrated that machine learning can dramatically extend evolution predictions of chaotic systems. The research is being described as "groundbreaking". What is your opinion about that?
What the paper says is that machine learning can tell you with higher accuracy what the state of the system is. If I know the initial conditions of the system more accurately, I can predict how it will evolve over a longer time. Let’s take the example of the billiard again: if I know exactly the initial conditions of the ball, I know where it will bounce; but after that I have no clue about what the interaction with other balls will cause. Machine learning is getting the initial state more accurate. And it’s surprising the level of precision they can get, it’s a truly remarkable result.
What about data science? Is that the most promising field in chaos research? What are we going to get from this kind of research and through this type of tool?
What’s promising? One thing about chaos theory is that you don’t know what’s going to be next. Even machine learning is not something people would have predicted. The most interesting areas of science to me are where I don’t know what’s going to happen next. Being a chaos theorist, I don’t like to predict the future. What I often say is that people don’t really know what’s going on right now. A writer came up with an idea, about 30 years ago, that “there was no new science because all the big things in science you could trace back thirty years and things happening now are not significant. So, this is the end of science.” But It only took 30 years for these little ideas to propagate and we don’t know what these ideas are now. Worrying about predicting the future is much less important than predicting the present. Nowadays, we can’t predict the present. Until we can predict the present, we won’t predict the future either.
Can machine learning and data science work together? How?
Chaos works with everything. Everything works with chaos, pretty much. Machine learning and data science will be interactive and work together to describe extremely complex problems. Let’s think to the stock market, extremely complex and unstable. It’s really related to chaos but worse. It’s not predictable in the short run. Chaos is predictable in the short run but this is a high-dimensional chaos, unstable in many different directions. Even a tiny electric circuit we think we know everything about, it will still be unstable. High dimensional systems like the stock market, the weather, genomics, and so on will get more complicated.
-- Anna Lombardi