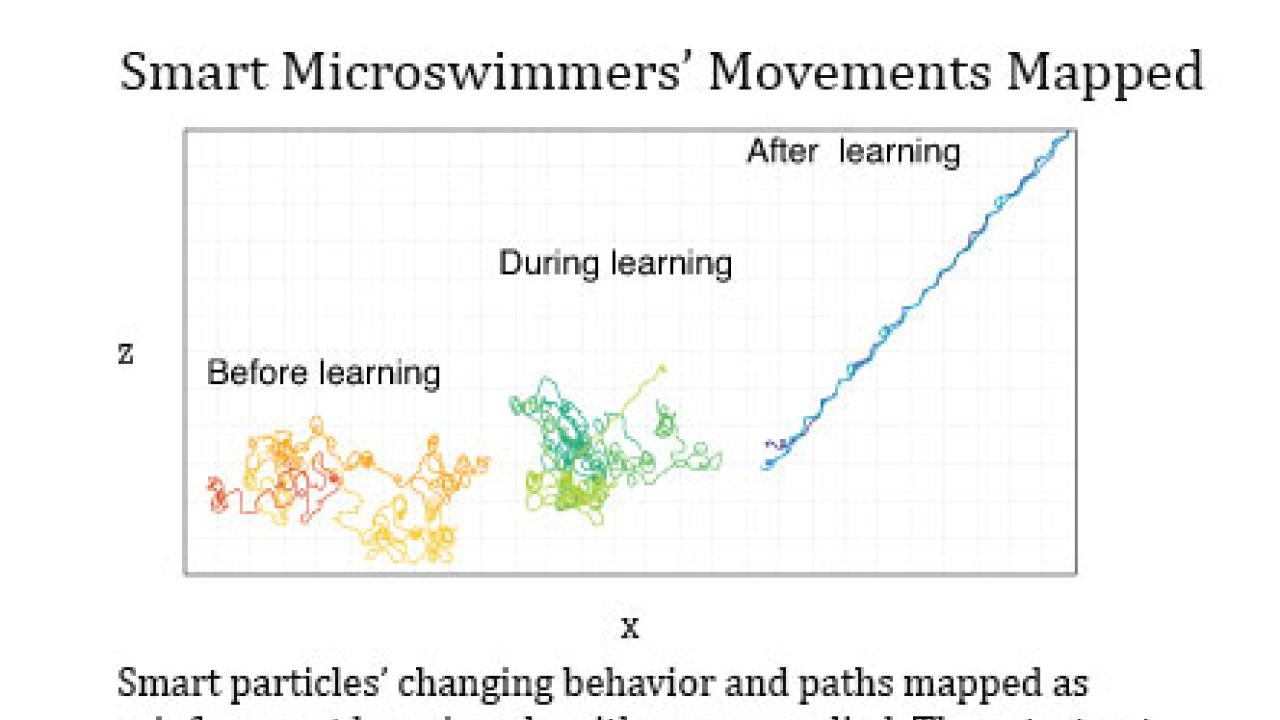
Tiny microorganisms usually seem pretty helpless, with few ways to move and even fewer ways to sense the world around them. But these microscopic creatures have survival goals like all others, and despite their few abilities, they have their own survival strategies. Now a group of physicists are studying these strategies to inform the engineering of artificial micro- and nano-swimmers, devices that could eventually be put to work delivering drugs or assessing machinery, as versatile tiny robots.
These microorganisms are any simple organism that has adapted to live in a fluid, including fresh water, sea water, or liquids inside of animals; ocean-dwelling phytoplankton and zooplankton are often studied. These organisms typically need to move to survive—a certain direction in a chemical gradient, like away from a hormone concentration, or upwards against gravity, to gain access to necessary nutrients in an ocean, or towards the light.
But the fluids where these microswimmers live is often dynamic, with complex flow patterns. And yet, these microorganisms still manage to move in their desired direction, even taking advantage of the flow whenever possible. ICTP physicist Antonio Celani and his collaborators set out to mimic these simple swimmers in a model, hoping their approaches could be applied to the engineering of smart swimming particles. It turns out that despite the little information the smart particles have, they can easily learn good strategies for achieving their goal. Their paper was published recently in Physical Review Letters, as an Editor's Suggestion.
The goal, in the simple model of a microswimmer the team started with, is to move upward in a fluid, against gravity, as far as possible. In calm waters, this theoretical smart swimmer can easily move upwards. But when the model incorporates the usual motion patterns of water in an ocean, the microswimmer can easily get trapped in circular flows, staying at one level for a long time. If it can make it out of the trap, it can also find 'elevator' regions, where water moves quickly upward. These elevators will take a microswimmer upwards and away from traps at one level—where it wants to go.
Taking a page from nature's microswimmers, Celani et al gave the model's microswimmer the ability to sense some basic cues from its environment. Microswimmers in nature use a variety of simple ways to try to influence where they go—they don't always just swim forward—so in the model, the smart particles can exert some influence over their direction of travel as well. The smart particles can sense very basic cues about their environment to make these decisions. With these tools, the goal is to find the best strategy for rising as far as possible up through the water.
It turns out that the framework known as reinforcement learning is very good at helping the microswimmers in the model construct efficient navigation strategies. Reinforcement learning is used widely in artificial intelligence and machine learning studies, and at its simplest, is a long process of interaction between the smart microswimmer and its environment, as the microswimmer learns by trial and error what's the best way to behave—in this case, navigate.
The good part of using reinforcement learning algorithms is that the mechanisms behind the dynamics of the environment and the ensuing behavior don't have to be completely understood or completely modeled for it to work. "You might be totally blind to how they might be operating," says Celani, "but you can still set a goal, measure performance and find ways to increase it." This works well for the model, and is a good sign for future engineers interested in making these microswimmers a reality. "On devices that small, you can't put on transmitters and remote controls," says Celani. But this paper shows that smart particles endowed with a reinforcement learning algorithm don't need to be micromanaged from afar—they can learn effective strategies for achieving their goal simply by interacting with their environment.
"The most interesting part of this result," says Celani, "is how little input is needed for the smart particles to successfully perform their task." Akin to nature, Celani's team didn't give the smart particles in the model much sense of what was happening around them. "The environment is very complex, and [microorganisms'] sensing is inherently limited. They have little memory, and can only take local measurements of flow and direction." But despite all this, they are still successful at evading traps in their journey upwards through the water. "It didn't take complicated neural networks for the microswimmers to perform this task." This is good news for all of the potential ways, imagined or yet to be, that microswimmers can be put to use in the future.
S. Colabrese, K. Gustavsson, A. Celani, and L. Biferale (2017) Flow Navigation by Smart Microswimmers via Reinforcement Learning Phys. Rev. Lett. 118, 158004
---- Kelsey Calhoun